Tackling Trust, Risk, and Security in AI Models: A Comprehensive Approach
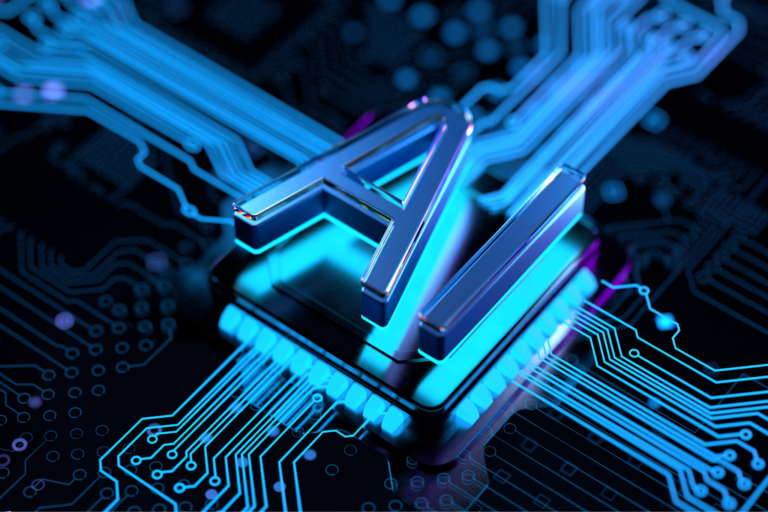
In the rapidly evolving world of artificial intelligence, the importance of establishing trust, managing risk, and ensuring security in AI models cannot be overstated. The introduction of AI Trust, Risk, and Security Management (AI TRiSM) represents a proactive solution aimed at addressing these critical concerns from the outset.
Big Picture: The Need for Upfront Integration of Governance
The integration of governance mechanisms upfront in the development and deployment of AI systems is essential. This approach ensures compliance, fairness, reliability, and data privacy, addressing risks that are often overlooked until AI models are operational. The complexity and opacity of AI systems necessitate a robust framework to mitigate potential adverse outcomes.
4 Pillars of AI TRiSM
- Explainability/Model Monitoring: The ability to clearly articulate the functionalities, strengths, weaknesses, biases, and expected behaviors of AI models to stakeholders is crucial. This transparency fosters trust and facilitates the identification and correction of biases and errors.
- ModelOps: Managing the risks associated with hosted generative AI applications requires specialized risk management processes. ModelOps emphasizes the operational aspects of deploying, monitoring, and maintaining AI models to ensure they remain accurate and effective over time.
- AI Application Security: The use of third-party AI tools introduces risks related to confidentiality breaches. Ensuring the security of AI applications is paramount to protect sensitive data and maintain user trust.
- Privacy: AI models are susceptible to adversarial attacks that can compromise privacy. Continuous monitoring and specialized controls are necessary to detect and prevent such attacks, safeguarding user data.
The Story Behind the Research
Insights from Avivah Litan, a Distinguished VP Analyst at Gartner, highlight the exponential risks organizations face without consistent management of AI risks. The potential for inaccurate, unethical, or unintended AI outcomes necessitates a comprehensive approach to AI risk management.
3 Key Points to Share
- AI TRiSM Capabilities: Implementing AI TRiSM capabilities is fundamental to ensuring the reliability, trustworthiness, security, and privacy of AI models. This framework supports better AI adoption outcomes, aligns with business goals, and enhances user acceptance.
- Drive Better Outcomes: By prioritizing AI TRiSM, organizations can effectively build protections into AI delivery and establish robust AI governance, driving improved outcomes and fostering innovation.
- Solution-Oriented Approach: Considering AI TRiSM as a set of solutions enables organizations to tackle the multifaceted challenges associated with AI models, from development through deployment.
Conclusion
The significance of AI TRiSM in mitigating risks and fostering trust, reliability, and compliance in AI applications is undeniable. Organizations are encouraged to prioritize the implementation of AI TRiSM strategies to safeguard against potential adverse outcomes and to ensure the ethical use of AI technologies. Embracing AI TRiSM is not just a risk management strategy—it is a foundational element for building trust and ensuring the responsible development and use of AI.