Can Machine Learning & AI Help Address the Tight Labor Market?
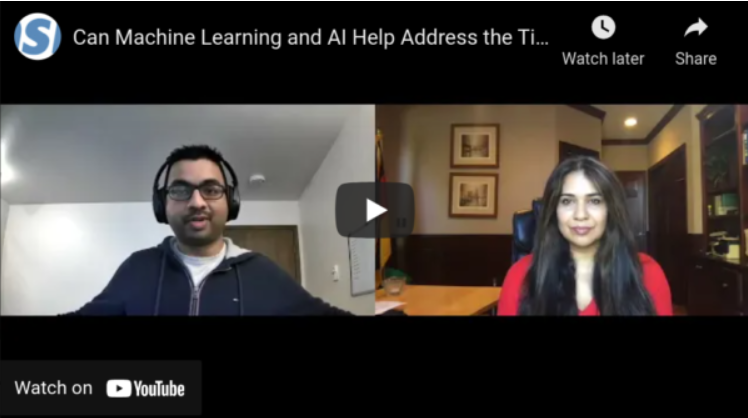
Softensity Leadership Series: A conversation with Varun Sridharan, Senior Technical Product Manager, Amazon Web Services Customer Behavior Analytics team.
By Softensity
Varun Sridharan is passionate about solving customers’ problems using machine learning and AI. He started his journey with machine learning back in 2014 and is on a mission to democratize the technology. Varun’s vision is nothing short of making machine learning and AI more accessible for the greater good of humanity. Softensity’s Monika Mueller sat down with Varun to talk about how companies can leverage the benefits of machine learning in a tight labor market.
Democratizing Machine Learning
We all know that machine learning is a type of artificial intelligence that allows software applications to become more accurate at predicting outcomes without being explicitly programmed to do so. And that machine learning algorithms use historical data inputs to predict new output values. Most of us also think that we need big data scientists to tap into the benefits of machine learning. Varun Sridharan suggests otherwise.
He points to a new technology that he has experience with: AutoML. This automated machine learning begins with data scientists, who build the right machine learning algorithms to the right hyperparameters. Then a company’s software engineers build the end-to-end pipeline to implement the technology. Theoretically, he says, you can put this technology into the hands of a company to build the machine learning models — both in the beginning, and to maintain over time.
Does this work for companies that don’t have a machine learning or data science background? Varun believes that a level of education and awareness is important to overcome this challenge and maintain realistic expectations. “Apart from that,” he says, “I see a great potential for AutoML in the future.”
Can Machine Learning Help Ease the IT Labor Shortage?
If organizations can begin to implement machine learning technologies without having to hire expensive data scientists, how can it be used to address the tight labor market? In the midst of the widespread labor shortages, hiring processes have never been more crucial. “Being able to attract the right talent, being able to screen the right talent, and then once they’re within your organization, being able to retain and support this talent is critically important,” says Monika. “So how can machine learning help us with these processes?”
Varun points to three stages of hiring where machine learning can help: pre-hiring, active hiring, and post-hiring. In the pre-hiring phase, organizations can use machine learning and AI to make staffing predictions based on the deals and projects in a company’s pipeline. This requires a good amount of historical data for accuracy. During the active hiring process, human emotions and reactions can be monitored to capture both the candidates’ and recruiter’s experience. After a hire has been made, a prescriptive report can be generated to provide insights that are tailored to an organization’s needs.
Just don’t forget to retrain your models, advises Varun. The more data your models acquire, the better they will be able to make predictions and generate a prescriptive report. Mueller agrees, echoing, “good data in equals good data out.”
How Can Organizations Begin to Implement Machine Learning?
When it comes to advice for technology leaders, Varun stresses the importance of realistic expectations. “Machine learning is not a magic wand,” he cautions. It’s important to first understand the technology’s capabilities. That starts with knowing the differences between the two broad categories of machine learning: artificial general intelligence (strong AI) and artificial narrow intelligence (weak AI).
Narrow artificial intelligence can perform constrained and targeted tasks, including automating time-consuming tasks by analyzing data better than humans. For example, staffing and recruiting needs rely on weak AI. Artificial general intelligence, on the other hand, is expected to perform like humans with a high level of cognitive ability. In Varun’s opinion, there is still a lot of progress to be made in this area.
“Having a very solid understanding of the difference between artificial narrow intelligence and artificial general intelligence is crucial in order to make an informed business decision for your business,” says Varun. Equally important, he says, is to “Have realistic expectations. Be pragmatic about it. Don’t overcommit — be incremental about it, and start with low expectations.”
Gain more insight by watching the entire conversation between Monika and Varun here.