Successes and Failures in AI Build vs. Buy Decisions
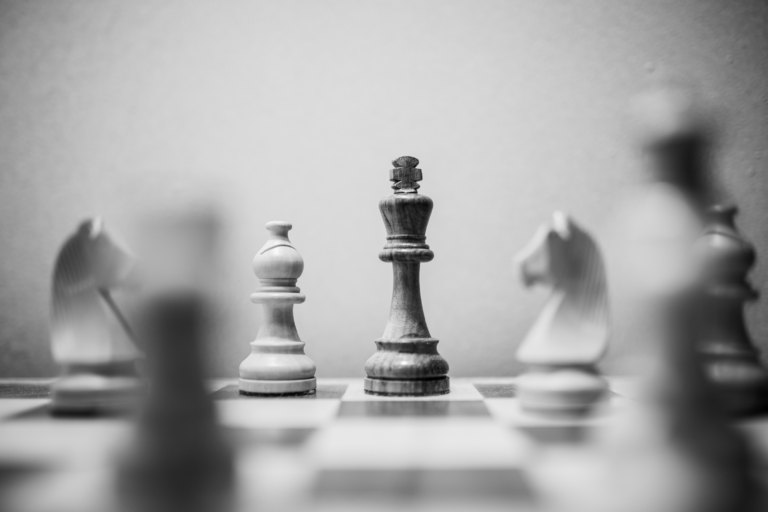
The AI Dilemma: Build or Buy?
The decision between building versus buying AI solutions presents a complex landscape, influenced by factors such as company size, industry, specific needs, and the technological landscape. Success stories across different companies demonstrate that the right approach can significantly influence outcomes, with considerations around data quality, technical complexity, privacy, security, ethical implications, and resistance to change playing crucial roles.
For instance, AI unicorns like Scale AI, Seismic, SenseTime, Shield AI, Sift, SigTuple, Sisense, and Skydio have showcased remarkable successes by building tailored AI solutions. These companies span various sectors from healthcare to sales enablement, drone technology, and fraud prevention, highlighting the diverse applications of AI across industries. The success factors for these AI startups include access to large and proprietary data sets, domain knowledge, and a deep talent pool around applied AI, which collectively enable them to provide valuable solutions to enterprises.
Success Factors and Challenges: Building a Tailored Solution
On the other hand, challenges such as ensuring data quality, managing technical complexity, and addressing privacy and security concerns are critical for successful AI implementation. Companies must carefully consider these challenges and address them through strategic planning and execution.
Researchers suggest that companies often dive into building their own AI solutions without considering more efficient options like buying off-the-shelf solutions or partnering with specialists. This can lead to AI sprawl, internal confusion, duplication of resources, and lack of alignment around core organizational goals, ultimately resulting in limited business impact. A well-thought-out AI strategy, choosing between building, buying, or partnering based on specific organizational needs and capabilities, is crucial for maximizing the value businesses can derive from AI.
Strategic Implementation: Maximizing Value from AI
In the manufacturing sector, AI has been instrumental in creating significant financial impacts through predictive maintenance, quality control, scrap reduction, yield optimization, and demand forecasting. Best practices for successful AI implementation in manufacturing include defining a suitable problem for AI to solve, involving the right people, and adopting a suitable process. Starting with a pilot project and scaling technology incrementally can be cost-effective and ensure a focus on ROI.
These insights underscore the importance of a strategic approach to AI deployment, whether through building, buying, or partnering. Companies must carefully assess their specific needs, capabilities, and the broader technological and competitive landscape to make decisions that best position them for success in leveraging AI.